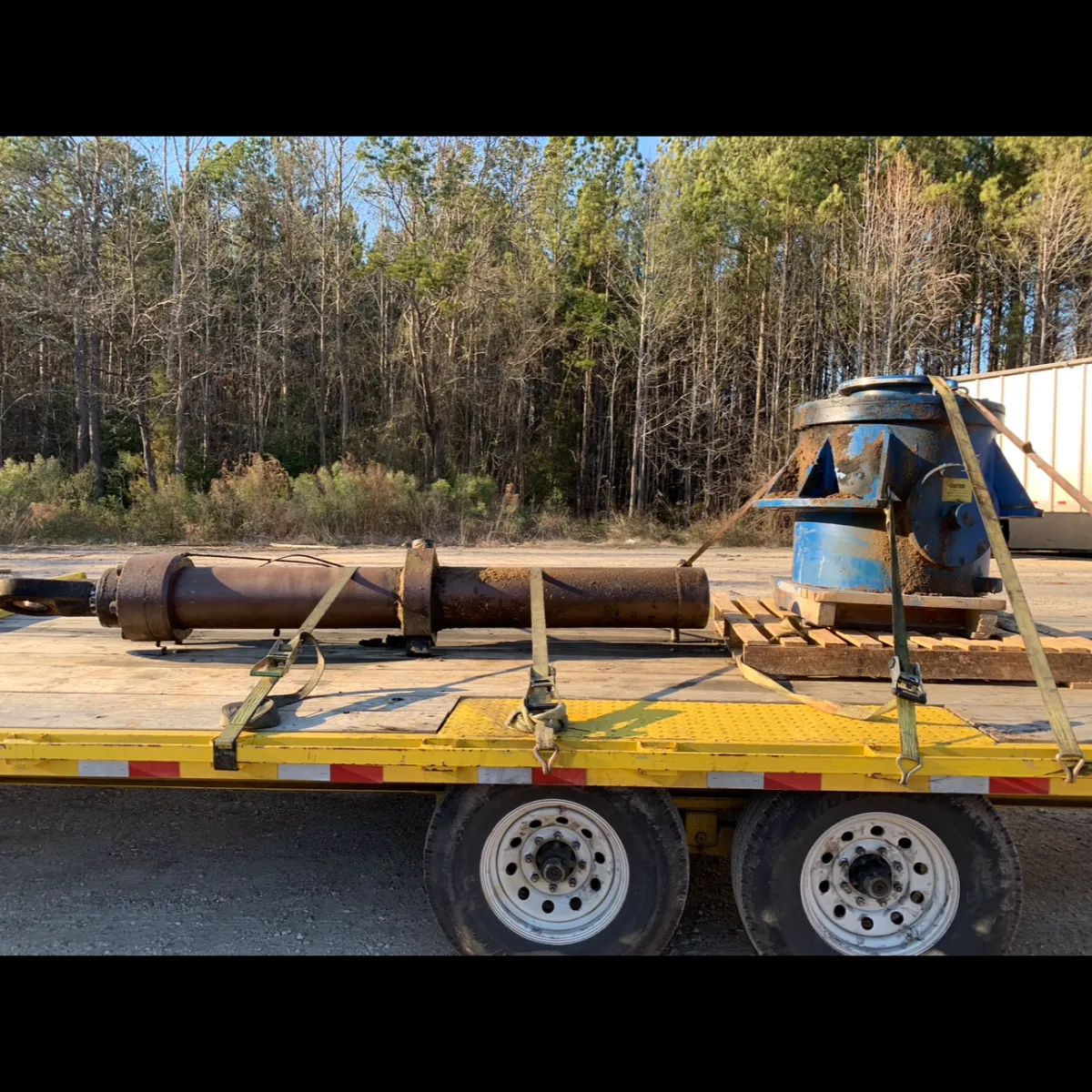
The Role of Predictive Analytics in Equipment Repairs
The Role of Predictive Analytics in Equipment Repairs
In today’s fast-paced industrial environments, unplanned downtime is one of the most costly risks businesses face. Whether you're in mining, construction, logistics, or manufacturing, equipment failure can bring operations to a halt. Fortunately, advancements in predictive analytics are transforming how we approach maintenance and repair.
Rather than waiting for equipment to break or relying solely on fixed schedules, companies now use predictive analyticsto detect early warning signs, forecast failures, and schedule repairs before problems escalate. In this article, we explore the powerful role predictive analytics plays in equipment repairs, and why it’s rapidly becoming a standard in modern maintenance strategies.
What Is Predictive Analytics?
Predictive analytics involves using historical data, real-time sensor inputs, and machine learning algorithms to forecast future outcomes. In the context of equipment maintenance, it means predicting when a component is likely to fail — before it actually does.
Key technologies involved:
Sensors and IoT (Internet of Things) devices
Telematics systems
Machine learning algorithms
CMMS (Computerized Maintenance Management Systems)
Cloud-based data analytics platforms
This data-driven approach empowers teams to make smarter, faster repair decisions with a higher degree of accuracy.
How Predictive Analytics Works in Equipment Maintenance
Data Collection
Sensors installed on equipment collect real-time data, such as temperature, vibration, fluid levels, RPMs, pressure, and usage hours.Data Integration
This information is sent to a centralized platform via telematics (e.g., Caterpillar Product Link, Komatsu KOMTRAX, or third-party tools).Pattern Recognition
Machine learning algorithms identify normal vs. abnormal patterns by analyzing historical data and operational baselines.Failure Prediction
When deviations from normal performance are detected, the system flags the component at risk and estimates a Time to Failure (TTF).Actionable Insights
Maintenance teams receive alerts and recommendations, allowing them to schedule a targeted repair or part replacement at the optimal time.
The Benefits of Predictive Analytics in Equipment Repairs
1. Reduced Unplanned Downtime
Predictive analytics allows teams to plan repairs during scheduled maintenance windows rather than reacting to breakdowns. This significantly reduces costly equipment downtime.
2. Lower Maintenance Costs
By identifying the exact part that needs attention, teams avoid unnecessary inspections, labor hours, and component replacements.
3. Extended Equipment Life
Timely repairs reduce strain on related systems and prevent cascading failures — extending the overall lifespan of your machinery.
4. Better Resource Planning
Technicians, spare parts, and tools can be coordinated in advance, improving efficiency and reducing the chaos of emergency callouts.
5. Data-Driven Decision Making
Instead of relying on gut instinct or visual checks alone, predictive analytics provides quantitative insight into machine health.
Common Applications in Predictive Equipment Repairs
Vibration Monitoring in Bearings and Rotating Equipment
Excessive vibration often precedes failure in motors, pumps, and gearboxes. Predictive tools flag anomalies early.Oil and Fluid Analysis
Changes in oil viscosity, contamination, or metal shavings in fluid samples can indicate internal wear.Thermal Imaging and Temperature Monitoring
Overheating components — like alternators or hydraulic valves — can be spotted and replaced before causing damage.Wear Monitoring for Undercarriages and Cutting Tools
Sensor-based measurement of wear helps determine the right time to change teeth, tracks, or cutting edges.Battery and Charging System Diagnostics
Voltage irregularities and cycle history are used to forecast electrical system issues before batteries fail.
Predictive Analytics vs. Preventive Maintenance
While both strategies reduce downtime, they differ in approach:
Preventive MaintenancePredictive AnalyticsTime or usage-basedData and condition-basedScheduled in advanceTriggered by real-time dataCan lead to over-maintenanceRepairs only when neededEasier to implementRequires tech investment
Best Practice: Many organizations use a hybrid model, where preventive maintenance is the baseline, and predictive analytics enhances precision.
Real-World Example: Mining Equipment
In the mining industry, predictive analytics is widely adopted to monitor haul trucks, excavators, crushers, and drills. For example:
A fleet of trucks equipped with vibration sensors detects abnormal axle behavior.
The system forecasts a potential bearing failure in Truck #217 within 60 hours.
The repair team schedules the truck for downtime during the next shift change.
Bearing is replaced before total failure — avoiding $30,000+ in parts, labor, and lost productivity.
This proactive approach keeps operations running, increases profitability, and improves safety.
Key Considerations for Implementation
1. Equipment Compatibility
Ensure your fleet supports sensors, telematics, or retrofitting options. Newer models often come equipped by default.
2. Data Quality
Garbage in = garbage out. Consistent, clean data is essential for effective predictive modeling.
3. Staff Training
Maintenance teams must be trained to interpret analytics reports, trust the data, and act on recommendations.
4. Integration with CMMS
Linking predictive analytics with your existing CMMS allows for automated work orders and better visibility.
5. ROI Tracking
Monitor key metrics like MTBF (Mean Time Between Failures), maintenance costs, and downtime hours to evaluate ROI.
Challenges of Predictive Analytics in Repairs
High Initial Setup Costs
Installing sensors, software, and analytics platforms requires investment.Connectivity Limitations
Remote job sites may struggle with internet access for real-time data transmission.Data Overload
Too much data without context can overwhelm technicians.False Positives
Algorithms must be tuned to avoid alert fatigue and unnecessary maintenance.
Despite these challenges, the long-term benefits far outweigh the upfront effort when implemented correctly.
Final Thoughts: Smarter Maintenance Starts with Prediction
Predictive analytics is changing the way we manage equipment repairs. It empowers companies to move from a reactive to a proactive mindset — one where equipment failure becomes the exception, not the norm.
As sensor technology improves and software becomes more accessible, predictive maintenance will continue to grow, especially in data-heavy sectors like mining, construction, and logistics.